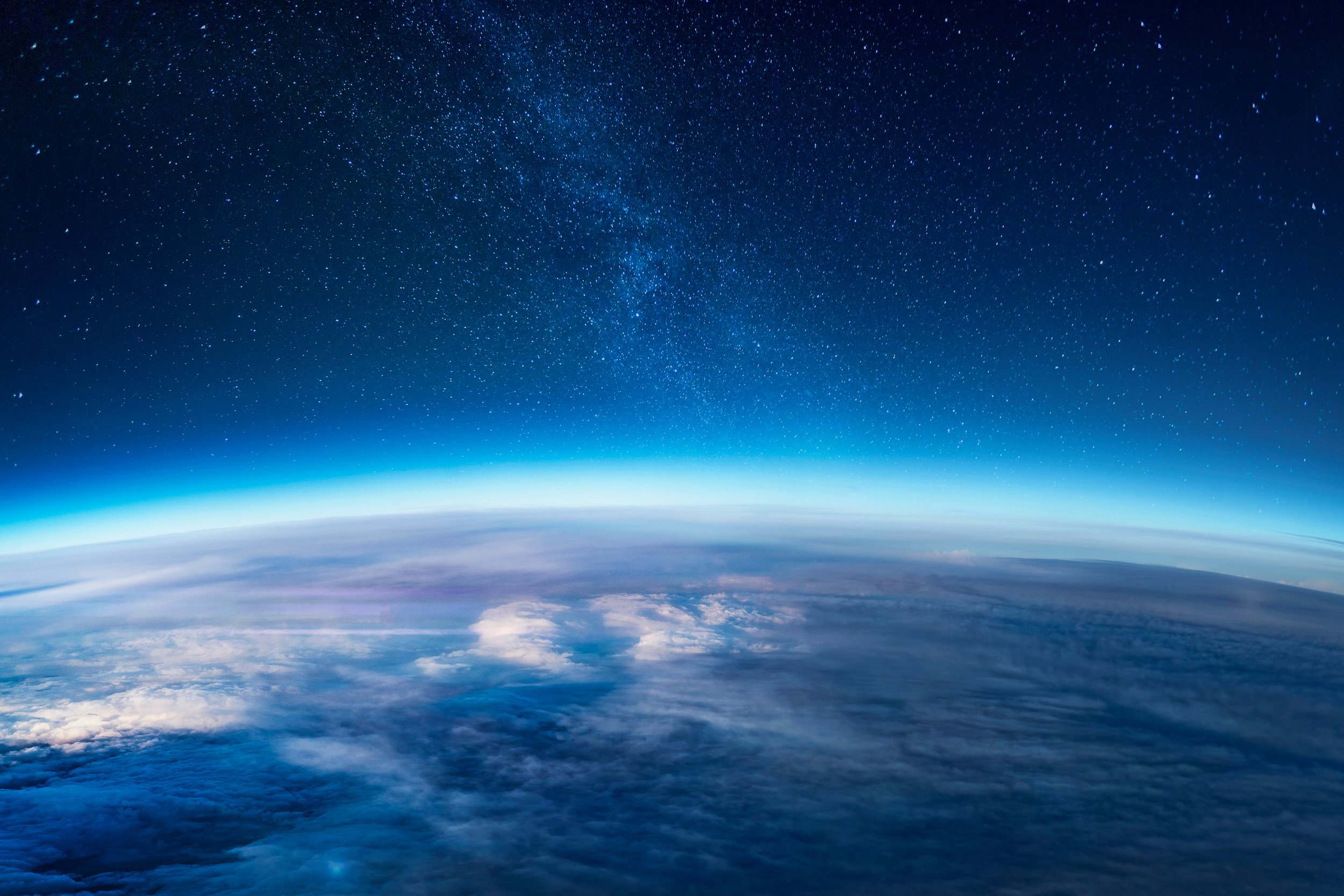
IMPACTS OF THE DIGITAL TRANSFROMATION
Impacts of The Digital Transformation
TO
Systems engineering environments fully leverage advances in digital technologies and modeling standards to enable rapid exploration of designs using high fidelity simulation, data visualization, and semantic web technologies. Systems engineering tools benefit from internet connectivity and knowledge representation to provide seamless exchange of information with other disciplines and their tool environments as part of a broader enterprise digital engineering environment. Further, systems engineers partner with machines to combine creativity and automation in a robust and agile design process.
FROM
Advancing digital technologies and standards development are enabling model-based systems engineering (MBSE) practices, but modeling- ecosystems are often rudimentary and incomplete.
Additionally, computation, cloud infrastructure, and data discovery are not being fully leveraged compared to other engineering and scientific disciplines.
Impacts of AI on Systems Engineering
TO
Systems engineering tools will be augmented with data driven and context aware algorithms that allow the systems engineer to focus more of their time on creative tasks and less on mundane tasks of data entry, consistency checking, report generation, and many others. Instead, systems
engineers provide their design intent and allow the tools to help guide them to high-quality specifications and design. Additionally systems engineers will themselves be more frequently designing systems with AI and ML components, requiring new systems engineering skills. The systems engineers will be critical in the development of data sets for algorithm training and testing, and will need to establish methods to validate systems behavior, performance and safety for an increasingly large number of systems with “learned” behaviors.
FROM
There is limited support in the current systems engineering tool ecosystem to help guide, evaluate, and optimize the specification, design, and analysis tasks the systems engineering team performs.
Entering data, building reports, and organizing diagrams are all manual efforts that detract from the value-added effort to create high quality system designs. Further, while the systems engineering community has contributed significant advancements in the development and verification of advanced algorithms, the systems engineering community has had limited engagement with the current exponential growth of AI and machine learning (ML) algorithms which are becoming increasingly ubiquitous in new systems.